How to read out the loss function in YOLO algorithm?
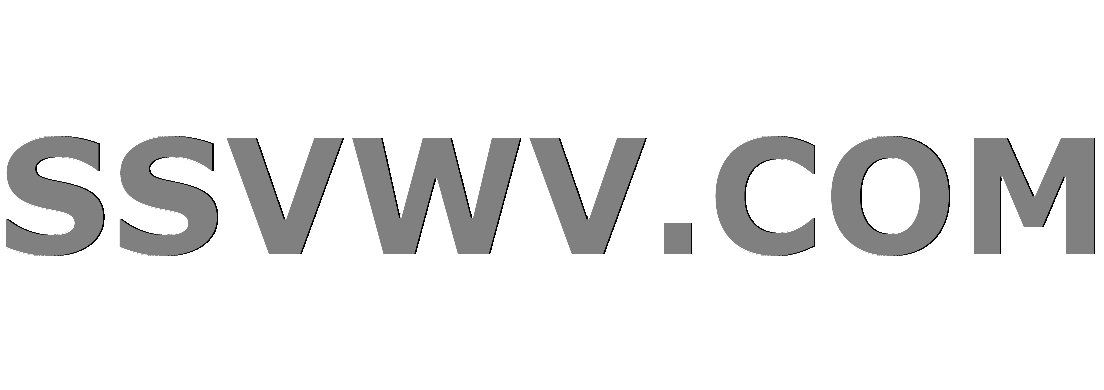
Multi tool use
Clash Royale CLAN TAG#URR8PPP
up vote
2
down vote
favorite
How do I read out the loss function used in YOLO?
I somehow need it for a class that I'm attending.
EDIT
Got an answer in Reddit!
optimization machine-learning
add a comment |Â
up vote
2
down vote
favorite
How do I read out the loss function used in YOLO?
I somehow need it for a class that I'm attending.
EDIT
Got an answer in Reddit!
optimization machine-learning
What do you mean by "Read out"? Do you just mean how to understand it?
– user3658307
Sep 8 at 2:44
@user3658307 Sorry about that, what I was trying to say was how do I read it out loud.
– Maning
Sep 9 at 13:06
add a comment |Â
up vote
2
down vote
favorite
up vote
2
down vote
favorite
How do I read out the loss function used in YOLO?
I somehow need it for a class that I'm attending.
EDIT
Got an answer in Reddit!
optimization machine-learning
How do I read out the loss function used in YOLO?
I somehow need it for a class that I'm attending.
EDIT
Got an answer in Reddit!
optimization machine-learning
optimization machine-learning
edited Sep 10 at 1:02
asked Sep 7 at 9:26


Maning
112
112
What do you mean by "Read out"? Do you just mean how to understand it?
– user3658307
Sep 8 at 2:44
@user3658307 Sorry about that, what I was trying to say was how do I read it out loud.
– Maning
Sep 9 at 13:06
add a comment |Â
What do you mean by "Read out"? Do you just mean how to understand it?
– user3658307
Sep 8 at 2:44
@user3658307 Sorry about that, what I was trying to say was how do I read it out loud.
– Maning
Sep 9 at 13:06
What do you mean by "Read out"? Do you just mean how to understand it?
– user3658307
Sep 8 at 2:44
What do you mean by "Read out"? Do you just mean how to understand it?
– user3658307
Sep 8 at 2:44
@user3658307 Sorry about that, what I was trying to say was how do I read it out loud.
– Maning
Sep 9 at 13:06
@user3658307 Sorry about that, what I was trying to say was how do I read it out loud.
– Maning
Sep 9 at 13:06
add a comment |Â
1 Answer
1
active
oldest
votes
up vote
0
down vote
It's a bit of an unexpected question, but I guess I would read it out by describing one term at a time. (Hopefully you meant a high-level description, not literally a phonetic sequence.) I'd say something like this when "reading it out":
Overall, we want to perform simultaneous object detection and classification. The indicator functions $(unicodex1D7D9_ij^ textobj )$ denote when the $j$th box in cell $i$ (i.e. the $j$th prediction has maximal confidence). Similarly the indicator $(unicodex1D7D9_i^ textobj )$ denotes whether there is an object in cell $i$.
Hatted quantities (e.g. $widehatx$, $widehatC$, $widehatp_i$) are predictions of their unhatted counterparts.
The sums over $i$ are over the gridded cells of the image, while the sums over $j$ iterate over the bounding box predictors (per cell).The first term checks that the predicted object box centers are close to the real ones, based on the squared distance between the centers.
The second term checks that the sizes (width $w$ and height $h$) of the predicted and true boxes are close to each other, to maximize overlap between them.
The third and fourth term measures the existence confidence (or objectness), i.e. $C_i$ gives the probability of an object being in cell $i$ at all, so the loss want the confidence of our learner to match whether or not an object is actually present.
The fifth term is the classification loss, so that the network correctly categorizes each object if an object exists there.
Might be helpful to look at other Yolo questions:
[1],
[2],
[3],
[4],
[5],
[6].
add a comment |Â
1 Answer
1
active
oldest
votes
1 Answer
1
active
oldest
votes
active
oldest
votes
active
oldest
votes
up vote
0
down vote
It's a bit of an unexpected question, but I guess I would read it out by describing one term at a time. (Hopefully you meant a high-level description, not literally a phonetic sequence.) I'd say something like this when "reading it out":
Overall, we want to perform simultaneous object detection and classification. The indicator functions $(unicodex1D7D9_ij^ textobj )$ denote when the $j$th box in cell $i$ (i.e. the $j$th prediction has maximal confidence). Similarly the indicator $(unicodex1D7D9_i^ textobj )$ denotes whether there is an object in cell $i$.
Hatted quantities (e.g. $widehatx$, $widehatC$, $widehatp_i$) are predictions of their unhatted counterparts.
The sums over $i$ are over the gridded cells of the image, while the sums over $j$ iterate over the bounding box predictors (per cell).The first term checks that the predicted object box centers are close to the real ones, based on the squared distance between the centers.
The second term checks that the sizes (width $w$ and height $h$) of the predicted and true boxes are close to each other, to maximize overlap between them.
The third and fourth term measures the existence confidence (or objectness), i.e. $C_i$ gives the probability of an object being in cell $i$ at all, so the loss want the confidence of our learner to match whether or not an object is actually present.
The fifth term is the classification loss, so that the network correctly categorizes each object if an object exists there.
Might be helpful to look at other Yolo questions:
[1],
[2],
[3],
[4],
[5],
[6].
add a comment |Â
up vote
0
down vote
It's a bit of an unexpected question, but I guess I would read it out by describing one term at a time. (Hopefully you meant a high-level description, not literally a phonetic sequence.) I'd say something like this when "reading it out":
Overall, we want to perform simultaneous object detection and classification. The indicator functions $(unicodex1D7D9_ij^ textobj )$ denote when the $j$th box in cell $i$ (i.e. the $j$th prediction has maximal confidence). Similarly the indicator $(unicodex1D7D9_i^ textobj )$ denotes whether there is an object in cell $i$.
Hatted quantities (e.g. $widehatx$, $widehatC$, $widehatp_i$) are predictions of their unhatted counterparts.
The sums over $i$ are over the gridded cells of the image, while the sums over $j$ iterate over the bounding box predictors (per cell).The first term checks that the predicted object box centers are close to the real ones, based on the squared distance between the centers.
The second term checks that the sizes (width $w$ and height $h$) of the predicted and true boxes are close to each other, to maximize overlap between them.
The third and fourth term measures the existence confidence (or objectness), i.e. $C_i$ gives the probability of an object being in cell $i$ at all, so the loss want the confidence of our learner to match whether or not an object is actually present.
The fifth term is the classification loss, so that the network correctly categorizes each object if an object exists there.
Might be helpful to look at other Yolo questions:
[1],
[2],
[3],
[4],
[5],
[6].
add a comment |Â
up vote
0
down vote
up vote
0
down vote
It's a bit of an unexpected question, but I guess I would read it out by describing one term at a time. (Hopefully you meant a high-level description, not literally a phonetic sequence.) I'd say something like this when "reading it out":
Overall, we want to perform simultaneous object detection and classification. The indicator functions $(unicodex1D7D9_ij^ textobj )$ denote when the $j$th box in cell $i$ (i.e. the $j$th prediction has maximal confidence). Similarly the indicator $(unicodex1D7D9_i^ textobj )$ denotes whether there is an object in cell $i$.
Hatted quantities (e.g. $widehatx$, $widehatC$, $widehatp_i$) are predictions of their unhatted counterparts.
The sums over $i$ are over the gridded cells of the image, while the sums over $j$ iterate over the bounding box predictors (per cell).The first term checks that the predicted object box centers are close to the real ones, based on the squared distance between the centers.
The second term checks that the sizes (width $w$ and height $h$) of the predicted and true boxes are close to each other, to maximize overlap between them.
The third and fourth term measures the existence confidence (or objectness), i.e. $C_i$ gives the probability of an object being in cell $i$ at all, so the loss want the confidence of our learner to match whether or not an object is actually present.
The fifth term is the classification loss, so that the network correctly categorizes each object if an object exists there.
Might be helpful to look at other Yolo questions:
[1],
[2],
[3],
[4],
[5],
[6].
It's a bit of an unexpected question, but I guess I would read it out by describing one term at a time. (Hopefully you meant a high-level description, not literally a phonetic sequence.) I'd say something like this when "reading it out":
Overall, we want to perform simultaneous object detection and classification. The indicator functions $(unicodex1D7D9_ij^ textobj )$ denote when the $j$th box in cell $i$ (i.e. the $j$th prediction has maximal confidence). Similarly the indicator $(unicodex1D7D9_i^ textobj )$ denotes whether there is an object in cell $i$.
Hatted quantities (e.g. $widehatx$, $widehatC$, $widehatp_i$) are predictions of their unhatted counterparts.
The sums over $i$ are over the gridded cells of the image, while the sums over $j$ iterate over the bounding box predictors (per cell).The first term checks that the predicted object box centers are close to the real ones, based on the squared distance between the centers.
The second term checks that the sizes (width $w$ and height $h$) of the predicted and true boxes are close to each other, to maximize overlap between them.
The third and fourth term measures the existence confidence (or objectness), i.e. $C_i$ gives the probability of an object being in cell $i$ at all, so the loss want the confidence of our learner to match whether or not an object is actually present.
The fifth term is the classification loss, so that the network correctly categorizes each object if an object exists there.
Might be helpful to look at other Yolo questions:
[1],
[2],
[3],
[4],
[5],
[6].
answered Sep 9 at 17:55
user3658307
4,3143945
4,3143945
add a comment |Â
add a comment |Â
Sign up or log in
StackExchange.ready(function ()
StackExchange.helpers.onClickDraftSave('#login-link');
);
Sign up using Google
Sign up using Facebook
Sign up using Email and Password
Post as a guest
StackExchange.ready(
function ()
StackExchange.openid.initPostLogin('.new-post-login', 'https%3a%2f%2fmath.stackexchange.com%2fquestions%2f2908437%2fhow-to-read-out-the-loss-function-in-yolo-algorithm%23new-answer', 'question_page');
);
Post as a guest
Sign up or log in
StackExchange.ready(function ()
StackExchange.helpers.onClickDraftSave('#login-link');
);
Sign up using Google
Sign up using Facebook
Sign up using Email and Password
Post as a guest
Sign up or log in
StackExchange.ready(function ()
StackExchange.helpers.onClickDraftSave('#login-link');
);
Sign up using Google
Sign up using Facebook
Sign up using Email and Password
Post as a guest
Sign up or log in
StackExchange.ready(function ()
StackExchange.helpers.onClickDraftSave('#login-link');
);
Sign up using Google
Sign up using Facebook
Sign up using Email and Password
Sign up using Google
Sign up using Facebook
Sign up using Email and Password
What do you mean by "Read out"? Do you just mean how to understand it?
– user3658307
Sep 8 at 2:44
@user3658307 Sorry about that, what I was trying to say was how do I read it out loud.
– Maning
Sep 9 at 13:06