Bounding coefficients of a uniform unit vector projected in a basis
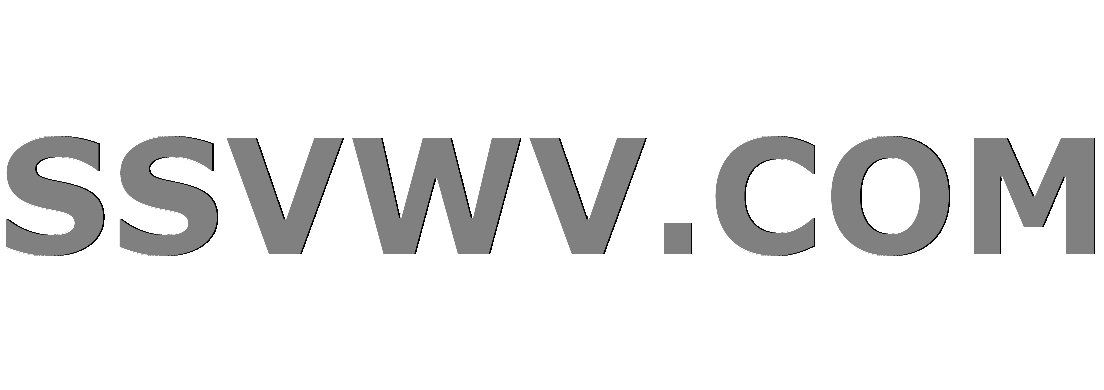
Multi tool use
Clash Royale CLAN TAG#URR8PPP
up vote
0
down vote
favorite
When bounding the convergence rate of many numerical linear algebra algorithms (e.g. the Lanczos method), typically one must use a bound on the coefficients of the initial vector in the eigenbasis of the input matrix. I wonder how to prove such a general bound for a uniformly randomly drawn vector from the unit sphere.
My idea so far is the following and I am wondering whether it makes sense:
Given a vector $v in mathbbR^n$ which is drawn random at uniform from the unit sphere, and some orthonormal basis $u_1,ldots,u_n$, I decompose this unit vector in this basis, i.e., $v = sum_i alpha_i u_i$. The question is now, whether I can lower bound the magnitude of these coefficients in probability, say with $p geq 1/2$, $alpha_i geq xi$.
My approach is the following very simple one:
Let $x$ be the vector with entries $alpha_i$;
- It is straight forward to bound, based on Gaussian concentration bounds the magnitude of the norm of $v$ in high probability.
- I could then conditionally on the norm being bounded find a lower bound on the entires, which are $chi^2$-distributed. However, I was not able to show a lower bound, since in particular I believe that the mean is $0$, which seems to imply that I can only obtain results of the form
$mathbb P(min_i X_i > t) leq sum_i=1^n mathbb P(X_i > t) leq n cdot exp(-t^2/2 sigma^2)$ and then using that
$min_1 leq i leq n |X_i| = min_1 leq i leq 2n X_i$ for $X_i+n = - X_i$ and hence obtaining:
$$mathbb P(min_i |X_i| > t) leq 2n cdot exp(-t^2/2 sigma^2).$$
However I would need in step three a different bound which lower bounds the probability. - If point (2) can be obtained, then I could just obtain the final success probability in terms of the error rates of the step (1,2) by bounding $mathbbP[v_i/||v|| geq c/sqrtn] geq 1 - delta_1 - delta_2$, where $delta_1/2$ are the results from the first two steps.
I was wondering if this approach is correct or if there is a better way?
Furthermore does anyone have a hint where I can find a lower bound on the minimum of a sub-gaussian distributed variable?
probability probability-distributions norm
add a comment |Â
up vote
0
down vote
favorite
When bounding the convergence rate of many numerical linear algebra algorithms (e.g. the Lanczos method), typically one must use a bound on the coefficients of the initial vector in the eigenbasis of the input matrix. I wonder how to prove such a general bound for a uniformly randomly drawn vector from the unit sphere.
My idea so far is the following and I am wondering whether it makes sense:
Given a vector $v in mathbbR^n$ which is drawn random at uniform from the unit sphere, and some orthonormal basis $u_1,ldots,u_n$, I decompose this unit vector in this basis, i.e., $v = sum_i alpha_i u_i$. The question is now, whether I can lower bound the magnitude of these coefficients in probability, say with $p geq 1/2$, $alpha_i geq xi$.
My approach is the following very simple one:
Let $x$ be the vector with entries $alpha_i$;
- It is straight forward to bound, based on Gaussian concentration bounds the magnitude of the norm of $v$ in high probability.
- I could then conditionally on the norm being bounded find a lower bound on the entires, which are $chi^2$-distributed. However, I was not able to show a lower bound, since in particular I believe that the mean is $0$, which seems to imply that I can only obtain results of the form
$mathbb P(min_i X_i > t) leq sum_i=1^n mathbb P(X_i > t) leq n cdot exp(-t^2/2 sigma^2)$ and then using that
$min_1 leq i leq n |X_i| = min_1 leq i leq 2n X_i$ for $X_i+n = - X_i$ and hence obtaining:
$$mathbb P(min_i |X_i| > t) leq 2n cdot exp(-t^2/2 sigma^2).$$
However I would need in step three a different bound which lower bounds the probability. - If point (2) can be obtained, then I could just obtain the final success probability in terms of the error rates of the step (1,2) by bounding $mathbbP[v_i/||v|| geq c/sqrtn] geq 1 - delta_1 - delta_2$, where $delta_1/2$ are the results from the first two steps.
I was wondering if this approach is correct or if there is a better way?
Furthermore does anyone have a hint where I can find a lower bound on the minimum of a sub-gaussian distributed variable?
probability probability-distributions norm
add a comment |Â
up vote
0
down vote
favorite
up vote
0
down vote
favorite
When bounding the convergence rate of many numerical linear algebra algorithms (e.g. the Lanczos method), typically one must use a bound on the coefficients of the initial vector in the eigenbasis of the input matrix. I wonder how to prove such a general bound for a uniformly randomly drawn vector from the unit sphere.
My idea so far is the following and I am wondering whether it makes sense:
Given a vector $v in mathbbR^n$ which is drawn random at uniform from the unit sphere, and some orthonormal basis $u_1,ldots,u_n$, I decompose this unit vector in this basis, i.e., $v = sum_i alpha_i u_i$. The question is now, whether I can lower bound the magnitude of these coefficients in probability, say with $p geq 1/2$, $alpha_i geq xi$.
My approach is the following very simple one:
Let $x$ be the vector with entries $alpha_i$;
- It is straight forward to bound, based on Gaussian concentration bounds the magnitude of the norm of $v$ in high probability.
- I could then conditionally on the norm being bounded find a lower bound on the entires, which are $chi^2$-distributed. However, I was not able to show a lower bound, since in particular I believe that the mean is $0$, which seems to imply that I can only obtain results of the form
$mathbb P(min_i X_i > t) leq sum_i=1^n mathbb P(X_i > t) leq n cdot exp(-t^2/2 sigma^2)$ and then using that
$min_1 leq i leq n |X_i| = min_1 leq i leq 2n X_i$ for $X_i+n = - X_i$ and hence obtaining:
$$mathbb P(min_i |X_i| > t) leq 2n cdot exp(-t^2/2 sigma^2).$$
However I would need in step three a different bound which lower bounds the probability. - If point (2) can be obtained, then I could just obtain the final success probability in terms of the error rates of the step (1,2) by bounding $mathbbP[v_i/||v|| geq c/sqrtn] geq 1 - delta_1 - delta_2$, where $delta_1/2$ are the results from the first two steps.
I was wondering if this approach is correct or if there is a better way?
Furthermore does anyone have a hint where I can find a lower bound on the minimum of a sub-gaussian distributed variable?
probability probability-distributions norm
When bounding the convergence rate of many numerical linear algebra algorithms (e.g. the Lanczos method), typically one must use a bound on the coefficients of the initial vector in the eigenbasis of the input matrix. I wonder how to prove such a general bound for a uniformly randomly drawn vector from the unit sphere.
My idea so far is the following and I am wondering whether it makes sense:
Given a vector $v in mathbbR^n$ which is drawn random at uniform from the unit sphere, and some orthonormal basis $u_1,ldots,u_n$, I decompose this unit vector in this basis, i.e., $v = sum_i alpha_i u_i$. The question is now, whether I can lower bound the magnitude of these coefficients in probability, say with $p geq 1/2$, $alpha_i geq xi$.
My approach is the following very simple one:
Let $x$ be the vector with entries $alpha_i$;
- It is straight forward to bound, based on Gaussian concentration bounds the magnitude of the norm of $v$ in high probability.
- I could then conditionally on the norm being bounded find a lower bound on the entires, which are $chi^2$-distributed. However, I was not able to show a lower bound, since in particular I believe that the mean is $0$, which seems to imply that I can only obtain results of the form
$mathbb P(min_i X_i > t) leq sum_i=1^n mathbb P(X_i > t) leq n cdot exp(-t^2/2 sigma^2)$ and then using that
$min_1 leq i leq n |X_i| = min_1 leq i leq 2n X_i$ for $X_i+n = - X_i$ and hence obtaining:
$$mathbb P(min_i |X_i| > t) leq 2n cdot exp(-t^2/2 sigma^2).$$
However I would need in step three a different bound which lower bounds the probability. - If point (2) can be obtained, then I could just obtain the final success probability in terms of the error rates of the step (1,2) by bounding $mathbbP[v_i/||v|| geq c/sqrtn] geq 1 - delta_1 - delta_2$, where $delta_1/2$ are the results from the first two steps.
I was wondering if this approach is correct or if there is a better way?
Furthermore does anyone have a hint where I can find a lower bound on the minimum of a sub-gaussian distributed variable?
probability probability-distributions norm
edited Aug 30 at 23:47
asked Aug 28 at 15:16
LeoW.
568
568
add a comment |Â
add a comment |Â
active
oldest
votes
active
oldest
votes
active
oldest
votes
active
oldest
votes
active
oldest
votes
Sign up or log in
StackExchange.ready(function ()
StackExchange.helpers.onClickDraftSave('#login-link');
);
Sign up using Google
Sign up using Facebook
Sign up using Email and Password
Post as a guest
StackExchange.ready(
function ()
StackExchange.openid.initPostLogin('.new-post-login', 'https%3a%2f%2fmath.stackexchange.com%2fquestions%2f2897384%2fbounding-coefficients-of-a-uniform-unit-vector-projected-in-a-basis%23new-answer', 'question_page');
);
Post as a guest
Sign up or log in
StackExchange.ready(function ()
StackExchange.helpers.onClickDraftSave('#login-link');
);
Sign up using Google
Sign up using Facebook
Sign up using Email and Password
Post as a guest
Sign up or log in
StackExchange.ready(function ()
StackExchange.helpers.onClickDraftSave('#login-link');
);
Sign up using Google
Sign up using Facebook
Sign up using Email and Password
Post as a guest
Sign up or log in
StackExchange.ready(function ()
StackExchange.helpers.onClickDraftSave('#login-link');
);
Sign up using Google
Sign up using Facebook
Sign up using Email and Password
Sign up using Google
Sign up using Facebook
Sign up using Email and Password