UMVUE of $fractheta1+theta$ and $frace^thetatheta$ from $U(-theta,theta)$ distribution
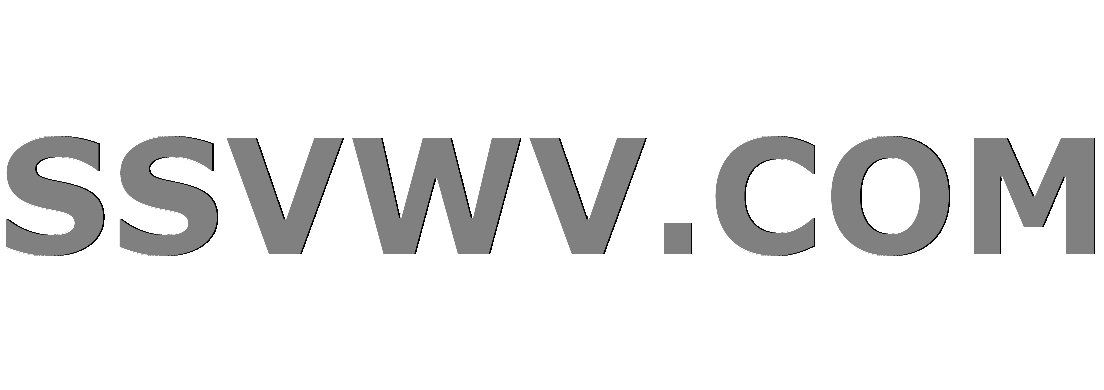
Multi tool use
Clash Royale CLAN TAG#URR8PPP
up vote
2
down vote
favorite
Let $X_1,X_2,dots, X_n$ be rvs with pdf:
$$f(xmid theta)=frac12thetaI(-theta<x<theta)$$
Find UMVUE of $(i)dfractheta1+theta$ and $(ii)dfrace^thetatheta$.
Note that, $(X_(1),X_(n))$ is complete sufficient statistic. But now I have to find unbiased estimator of $(i),(ii)$ of the form $g(X_(1),X_(n))$, then $g$ will become UMVUE. But I could not find such $g$. Thanks for any help.
I tried to find $E(X_(1)/X_(n))$, but it came out a total mess.
Here $X_(1)=min(X_1,X_2,dots, X_n)$ and $X_(n)=max(X_1,X_2,dots, X_n)$.
statistics probability-distributions statistical-inference order-statistics parameter-estimation
 |Â
show 1 more comment
up vote
2
down vote
favorite
Let $X_1,X_2,dots, X_n$ be rvs with pdf:
$$f(xmid theta)=frac12thetaI(-theta<x<theta)$$
Find UMVUE of $(i)dfractheta1+theta$ and $(ii)dfrace^thetatheta$.
Note that, $(X_(1),X_(n))$ is complete sufficient statistic. But now I have to find unbiased estimator of $(i),(ii)$ of the form $g(X_(1),X_(n))$, then $g$ will become UMVUE. But I could not find such $g$. Thanks for any help.
I tried to find $E(X_(1)/X_(n))$, but it came out a total mess.
Here $X_(1)=min(X_1,X_2,dots, X_n)$ and $X_(n)=max(X_1,X_2,dots, X_n)$.
statistics probability-distributions statistical-inference order-statistics parameter-estimation
1
A complete sufficient statistic for $theta$ is simply $max |X_i|$. Did you try finding the unbiased estimators of i) and ii) ?
– StubbornAtom
Aug 21 at 16:04
@StubbornAtom I wrote that I could not find u.e.. I am asking for a way (or a hint) to find u.e.
– Stat_prob_001
Aug 21 at 16:09
Regarding i), let $g(theta)=fractheta1+theta$, where $theta$ is obviously positive. If $theta>1$, then one could write $$g(theta)=left(1+frac1thetaright)^-1=1-frac1theta+frac1theta^2-frac1theta^3+cdots$$ If $0<theta<1$, then $$g(theta)=theta(1+theta+theta^2+cdots)$$...
– StubbornAtom
Aug 21 at 17:11
...So if one could find unbiased estimators of the form $theta^k$ or $1/theta^k$, then combining them he could get an unbiased estimator $T$ (say) of $g(theta)$. By Lehmann-Scheffe theorem, $E(Tmid max|X_i|)$ would be the UMVUE of $g(theta)$. Note that $X_isim U(-theta,theta)implies|X_i|sim U(0,theta)$ and $max |X_i|$ is a complete sufficient statistic for the family. This is just a thought, since ultimately an unbiased estimator of $g(theta)$ based on $max |X_i|$ would be enough for the final answer.
– StubbornAtom
Aug 21 at 17:11
@StubbornAtom very much thank you. Although I could not find any estimator of $1/theta^k$, for $kgeq n$. Is it even possible to find unbiased estimator??
– Stat_prob_001
Aug 21 at 18:53
 |Â
show 1 more comment
up vote
2
down vote
favorite
up vote
2
down vote
favorite
Let $X_1,X_2,dots, X_n$ be rvs with pdf:
$$f(xmid theta)=frac12thetaI(-theta<x<theta)$$
Find UMVUE of $(i)dfractheta1+theta$ and $(ii)dfrace^thetatheta$.
Note that, $(X_(1),X_(n))$ is complete sufficient statistic. But now I have to find unbiased estimator of $(i),(ii)$ of the form $g(X_(1),X_(n))$, then $g$ will become UMVUE. But I could not find such $g$. Thanks for any help.
I tried to find $E(X_(1)/X_(n))$, but it came out a total mess.
Here $X_(1)=min(X_1,X_2,dots, X_n)$ and $X_(n)=max(X_1,X_2,dots, X_n)$.
statistics probability-distributions statistical-inference order-statistics parameter-estimation
Let $X_1,X_2,dots, X_n$ be rvs with pdf:
$$f(xmid theta)=frac12thetaI(-theta<x<theta)$$
Find UMVUE of $(i)dfractheta1+theta$ and $(ii)dfrace^thetatheta$.
Note that, $(X_(1),X_(n))$ is complete sufficient statistic. But now I have to find unbiased estimator of $(i),(ii)$ of the form $g(X_(1),X_(n))$, then $g$ will become UMVUE. But I could not find such $g$. Thanks for any help.
I tried to find $E(X_(1)/X_(n))$, but it came out a total mess.
Here $X_(1)=min(X_1,X_2,dots, X_n)$ and $X_(n)=max(X_1,X_2,dots, X_n)$.
statistics probability-distributions statistical-inference order-statistics parameter-estimation
edited Aug 24 at 18:12
Michael Hardy
205k23187463
205k23187463
asked Aug 21 at 14:21


Stat_prob_001
316110
316110
1
A complete sufficient statistic for $theta$ is simply $max |X_i|$. Did you try finding the unbiased estimators of i) and ii) ?
– StubbornAtom
Aug 21 at 16:04
@StubbornAtom I wrote that I could not find u.e.. I am asking for a way (or a hint) to find u.e.
– Stat_prob_001
Aug 21 at 16:09
Regarding i), let $g(theta)=fractheta1+theta$, where $theta$ is obviously positive. If $theta>1$, then one could write $$g(theta)=left(1+frac1thetaright)^-1=1-frac1theta+frac1theta^2-frac1theta^3+cdots$$ If $0<theta<1$, then $$g(theta)=theta(1+theta+theta^2+cdots)$$...
– StubbornAtom
Aug 21 at 17:11
...So if one could find unbiased estimators of the form $theta^k$ or $1/theta^k$, then combining them he could get an unbiased estimator $T$ (say) of $g(theta)$. By Lehmann-Scheffe theorem, $E(Tmid max|X_i|)$ would be the UMVUE of $g(theta)$. Note that $X_isim U(-theta,theta)implies|X_i|sim U(0,theta)$ and $max |X_i|$ is a complete sufficient statistic for the family. This is just a thought, since ultimately an unbiased estimator of $g(theta)$ based on $max |X_i|$ would be enough for the final answer.
– StubbornAtom
Aug 21 at 17:11
@StubbornAtom very much thank you. Although I could not find any estimator of $1/theta^k$, for $kgeq n$. Is it even possible to find unbiased estimator??
– Stat_prob_001
Aug 21 at 18:53
 |Â
show 1 more comment
1
A complete sufficient statistic for $theta$ is simply $max |X_i|$. Did you try finding the unbiased estimators of i) and ii) ?
– StubbornAtom
Aug 21 at 16:04
@StubbornAtom I wrote that I could not find u.e.. I am asking for a way (or a hint) to find u.e.
– Stat_prob_001
Aug 21 at 16:09
Regarding i), let $g(theta)=fractheta1+theta$, where $theta$ is obviously positive. If $theta>1$, then one could write $$g(theta)=left(1+frac1thetaright)^-1=1-frac1theta+frac1theta^2-frac1theta^3+cdots$$ If $0<theta<1$, then $$g(theta)=theta(1+theta+theta^2+cdots)$$...
– StubbornAtom
Aug 21 at 17:11
...So if one could find unbiased estimators of the form $theta^k$ or $1/theta^k$, then combining them he could get an unbiased estimator $T$ (say) of $g(theta)$. By Lehmann-Scheffe theorem, $E(Tmid max|X_i|)$ would be the UMVUE of $g(theta)$. Note that $X_isim U(-theta,theta)implies|X_i|sim U(0,theta)$ and $max |X_i|$ is a complete sufficient statistic for the family. This is just a thought, since ultimately an unbiased estimator of $g(theta)$ based on $max |X_i|$ would be enough for the final answer.
– StubbornAtom
Aug 21 at 17:11
@StubbornAtom very much thank you. Although I could not find any estimator of $1/theta^k$, for $kgeq n$. Is it even possible to find unbiased estimator??
– Stat_prob_001
Aug 21 at 18:53
1
1
A complete sufficient statistic for $theta$ is simply $max |X_i|$. Did you try finding the unbiased estimators of i) and ii) ?
– StubbornAtom
Aug 21 at 16:04
A complete sufficient statistic for $theta$ is simply $max |X_i|$. Did you try finding the unbiased estimators of i) and ii) ?
– StubbornAtom
Aug 21 at 16:04
@StubbornAtom I wrote that I could not find u.e.. I am asking for a way (or a hint) to find u.e.
– Stat_prob_001
Aug 21 at 16:09
@StubbornAtom I wrote that I could not find u.e.. I am asking for a way (or a hint) to find u.e.
– Stat_prob_001
Aug 21 at 16:09
Regarding i), let $g(theta)=fractheta1+theta$, where $theta$ is obviously positive. If $theta>1$, then one could write $$g(theta)=left(1+frac1thetaright)^-1=1-frac1theta+frac1theta^2-frac1theta^3+cdots$$ If $0<theta<1$, then $$g(theta)=theta(1+theta+theta^2+cdots)$$...
– StubbornAtom
Aug 21 at 17:11
Regarding i), let $g(theta)=fractheta1+theta$, where $theta$ is obviously positive. If $theta>1$, then one could write $$g(theta)=left(1+frac1thetaright)^-1=1-frac1theta+frac1theta^2-frac1theta^3+cdots$$ If $0<theta<1$, then $$g(theta)=theta(1+theta+theta^2+cdots)$$...
– StubbornAtom
Aug 21 at 17:11
...So if one could find unbiased estimators of the form $theta^k$ or $1/theta^k$, then combining them he could get an unbiased estimator $T$ (say) of $g(theta)$. By Lehmann-Scheffe theorem, $E(Tmid max|X_i|)$ would be the UMVUE of $g(theta)$. Note that $X_isim U(-theta,theta)implies|X_i|sim U(0,theta)$ and $max |X_i|$ is a complete sufficient statistic for the family. This is just a thought, since ultimately an unbiased estimator of $g(theta)$ based on $max |X_i|$ would be enough for the final answer.
– StubbornAtom
Aug 21 at 17:11
...So if one could find unbiased estimators of the form $theta^k$ or $1/theta^k$, then combining them he could get an unbiased estimator $T$ (say) of $g(theta)$. By Lehmann-Scheffe theorem, $E(Tmid max|X_i|)$ would be the UMVUE of $g(theta)$. Note that $X_isim U(-theta,theta)implies|X_i|sim U(0,theta)$ and $max |X_i|$ is a complete sufficient statistic for the family. This is just a thought, since ultimately an unbiased estimator of $g(theta)$ based on $max |X_i|$ would be enough for the final answer.
– StubbornAtom
Aug 21 at 17:11
@StubbornAtom very much thank you. Although I could not find any estimator of $1/theta^k$, for $kgeq n$. Is it even possible to find unbiased estimator??
– Stat_prob_001
Aug 21 at 18:53
@StubbornAtom very much thank you. Although I could not find any estimator of $1/theta^k$, for $kgeq n$. Is it even possible to find unbiased estimator??
– Stat_prob_001
Aug 21 at 18:53
 |Â
show 1 more comment
1 Answer
1
active
oldest
votes
up vote
5
down vote
accepted
You have a $U(-theta,theta)$ population where $thetainmathbb R^+$.
Joint density of the sample $mathbf X=(X_1,X_2,ldots,X_n)$ is
beginalign
f_theta(mathbf x)&=frac1(2theta)^nmathbf1_-theta < x_1, ldots, x_n < theta
\&=frac1(2theta)^nmathbf1_x_1
\&=frac1(2theta)^nmathbf1_max_1le ile n
endalign
It is clear from Factorization theorem that a sufficient statistic for $theta$ is $$T(mathbf X)=max_1le ile n|X_i|$$
One could verify that $|X_i|sim U(0,theta)$, so that the density of $T$ is $$g_theta(t)=fracntheta^nt^n-1mathbf1_0<t<theta$$
That $T$ is a complete statistic for $theta$ is well-known.
We simply have to find unbiased estimators of the parametric functions of $theta$ based on the complete sufficient statistic. This would give us the UMVUE by the Lehmann-Scheffe theorem.
As the support of the complete sufficient statistic here depends on the parameter $theta$, unbiased estimators can be directly obtained through differentiation.
Let $h_1(T)$ and $h_2(T)$ be unbiased estimators of $theta/(1+theta)$ and $e^theta/theta$ respectively, based on the complete sufficient statistic $T$.
That is, for all $theta>0$,
beginalign
qquadquadfracntheta^nint_0^thetah_1(t)t^n-1,dt&=fractheta1+theta
\implies int_0^thetah_1(t)t^n-1,dt &= fractheta^n+1n(1+theta)
endalign
Differentiating both sides wrt $theta$,
beginalign
h_1(theta)theta^n-1&=fractheta^n(ntheta+n+1)n(1+theta)^2
\implies h_1(theta) &=fractheta(ntheta+n+1)n(1+theta)^2
endalign
Hence, $$h_1(T)=fracT(nT+n+1)n(1+T)^2$$
Similarly for the second problem, for all $theta>0$,
beginalign
qquadquadfracntheta^nint_0^thetah_2(t)t^n-1,dt&=frace^thetatheta
\implies int_0^thetah_2(t)t^n-1,dt &= fractheta^n-1 e^thetan
endalign
Differentiating both sides wrt $theta$ yields
beginalign
h_2(theta)theta^n-1&=frace^thetatheta^n-2(theta+n-1)n
\implies h_2(theta) &=frace^theta(theta+n-1)ntheta
endalign
So, $$h_2(T)=frace^T(T+n-1)nT$$
In my initial answer, the following calculation for the UMVUE was rather unnecessary and complicated. Had the support not depended on the parameter, I might have tried this. I am keeping this part in the answer as I might be able to salvage the somewhat faulty argument on some further consideration :
For $k> -n$, we have
beginalign
E_theta(T^k)&=fracntheta^nint_0^theta t^k+n-1,dt\[8pt]
& = fracntheta^kn+k
endalign
This suggests that an unbiased estimator of $theta^k$ based on $T$ is $$left(fracn+knright)T^k$$
For the first problem, one could write
beginalign
fractheta1+theta&=
begincasesleft(1+frac1thetaright)^-1=1-frac1theta+frac1theta^2-frac1theta^3+cdots&,text if theta>1\\theta(1+theta+theta^2+cdots)&,text if 0<theta<1endcases
endalign
For $0<theta<1$, we have
$$E_thetaleft[left(fracn+1nright)T+left(fracn+2nright)T^2+cdotsright]=theta+theta^2+cdots$$
Or, $$E_thetaleft[sum_k=1^inftyleft(fracn+knright)T^kright]=fractheta1+theta$$
For $theta>1$,
$$E_thetaleft[1-left(fracn-1nright)frac1T+left(fracn-2nright)frac1T^2-cdotsright]=1-frac1theta+frac1theta^2-cdots$$
That is, $$E_thetaleft[sum_k=0^inftyleft(fracn-knright)frac(-1)^kT^kright]=fractheta1+theta$$
Hence by Lehmann-Scheffe theorem, UMVUE of $theta/(1+theta)$ is
beginalign
h_1(T)&=begincasesdisplaystylesum_k=1^inftyleft(fracn+knright)T^k&,text if 0<theta<1\\displaystylesum_k=0^inftyleft(fracn-knright)frac(-1)^kT^k&,text if thetage1 endcases
\\&=begincasesdisplaystylefracT(n+1-nT)n(T-1)^2&,text if 0<theta<1\\displaystylefracT(n+1+nT)n(T+1)^2&,text if thetage1endcases
endalign
However, upon verification of unbiasedness for some values of $n$, it looks like only $$h_1(T)=displaystylefracT(n+1+nT)n(T+1)^2$$ should be the correct answer for all $theta>0$. I am not quite sure why that happens.
For the second problem, we can use the power series expansion of $e^theta$ to obtain
$$E_thetaleft[sum_k=-1^inftyleft(fracn+knright)fracT^k(k+1)!right]=sum_j=0^infty fractheta^j-1j!=frace^thetatheta$$
So the UMVUE of $e^theta/theta$ is
beginalign
h_2(T)&=sum_k=-1^inftyleft(fracn+knright)fracT^k(k+1)!
\\&=frace^T(n-1+T)nT
endalign
1
ah! I understand! But the problem I am getting is somewhere else. Take $k=-n-2$. Then: $$E(T^-n-2)=fracntheta^nint_0^thetat^-n-2+n-1dt=fracntheta^nint_0^thetat^-3dt=fracntheta^nint_0^thetafrac1t^3dt$$ I can not integrate this. That is the problem.
– Stat_prob_001
Aug 23 at 10:11
2
@StubbornAtom, I think the OP meant to say that the integration $int_0^theta frac1t^3dt$ is undefined at $0$, you can not integrate that thing in the first place.
– Shanks
Aug 23 at 16:11
1
@Stat_prob_001 Do have a look at my edit.
– StubbornAtom
Aug 24 at 17:48
1
@StubbornAtom thanks for the update. This is definitely a very useful method. But, just for curiosity, I tried to use the that method to find UMVUE of $theta^-n-2$, and it came out to be $-frac2nT^-n-2$. But $E(-frac2nT^-n-2)$ do not exists. So, I think (maybe), this method can only be used if UMVUE of a function $theta$ exists for all $theta$. So, it is needed to prove that UMVUE of $fractheta1+theta$ actually exists for all $theta$. Is there is any way to establish such think? I mean how to prove UMVUE of $fractheta1+theta$ exists?
– Stat_prob_001
Aug 25 at 8:12
1
@Stat_prob_001 There is a theorem that says a necessary and sufficient condition for an unbiased estimator to be the UMVUE of a given parametric function is that it must be uncorrelated with every unbiased estimator of zero. This result can be used to inspect whether UMVUE for a parametric function exists or not.
– StubbornAtom
Aug 25 at 8:26
 |Â
show 4 more comments
1 Answer
1
active
oldest
votes
1 Answer
1
active
oldest
votes
active
oldest
votes
active
oldest
votes
up vote
5
down vote
accepted
You have a $U(-theta,theta)$ population where $thetainmathbb R^+$.
Joint density of the sample $mathbf X=(X_1,X_2,ldots,X_n)$ is
beginalign
f_theta(mathbf x)&=frac1(2theta)^nmathbf1_-theta < x_1, ldots, x_n < theta
\&=frac1(2theta)^nmathbf1_x_1
\&=frac1(2theta)^nmathbf1_max_1le ile n
endalign
It is clear from Factorization theorem that a sufficient statistic for $theta$ is $$T(mathbf X)=max_1le ile n|X_i|$$
One could verify that $|X_i|sim U(0,theta)$, so that the density of $T$ is $$g_theta(t)=fracntheta^nt^n-1mathbf1_0<t<theta$$
That $T$ is a complete statistic for $theta$ is well-known.
We simply have to find unbiased estimators of the parametric functions of $theta$ based on the complete sufficient statistic. This would give us the UMVUE by the Lehmann-Scheffe theorem.
As the support of the complete sufficient statistic here depends on the parameter $theta$, unbiased estimators can be directly obtained through differentiation.
Let $h_1(T)$ and $h_2(T)$ be unbiased estimators of $theta/(1+theta)$ and $e^theta/theta$ respectively, based on the complete sufficient statistic $T$.
That is, for all $theta>0$,
beginalign
qquadquadfracntheta^nint_0^thetah_1(t)t^n-1,dt&=fractheta1+theta
\implies int_0^thetah_1(t)t^n-1,dt &= fractheta^n+1n(1+theta)
endalign
Differentiating both sides wrt $theta$,
beginalign
h_1(theta)theta^n-1&=fractheta^n(ntheta+n+1)n(1+theta)^2
\implies h_1(theta) &=fractheta(ntheta+n+1)n(1+theta)^2
endalign
Hence, $$h_1(T)=fracT(nT+n+1)n(1+T)^2$$
Similarly for the second problem, for all $theta>0$,
beginalign
qquadquadfracntheta^nint_0^thetah_2(t)t^n-1,dt&=frace^thetatheta
\implies int_0^thetah_2(t)t^n-1,dt &= fractheta^n-1 e^thetan
endalign
Differentiating both sides wrt $theta$ yields
beginalign
h_2(theta)theta^n-1&=frace^thetatheta^n-2(theta+n-1)n
\implies h_2(theta) &=frace^theta(theta+n-1)ntheta
endalign
So, $$h_2(T)=frace^T(T+n-1)nT$$
In my initial answer, the following calculation for the UMVUE was rather unnecessary and complicated. Had the support not depended on the parameter, I might have tried this. I am keeping this part in the answer as I might be able to salvage the somewhat faulty argument on some further consideration :
For $k> -n$, we have
beginalign
E_theta(T^k)&=fracntheta^nint_0^theta t^k+n-1,dt\[8pt]
& = fracntheta^kn+k
endalign
This suggests that an unbiased estimator of $theta^k$ based on $T$ is $$left(fracn+knright)T^k$$
For the first problem, one could write
beginalign
fractheta1+theta&=
begincasesleft(1+frac1thetaright)^-1=1-frac1theta+frac1theta^2-frac1theta^3+cdots&,text if theta>1\\theta(1+theta+theta^2+cdots)&,text if 0<theta<1endcases
endalign
For $0<theta<1$, we have
$$E_thetaleft[left(fracn+1nright)T+left(fracn+2nright)T^2+cdotsright]=theta+theta^2+cdots$$
Or, $$E_thetaleft[sum_k=1^inftyleft(fracn+knright)T^kright]=fractheta1+theta$$
For $theta>1$,
$$E_thetaleft[1-left(fracn-1nright)frac1T+left(fracn-2nright)frac1T^2-cdotsright]=1-frac1theta+frac1theta^2-cdots$$
That is, $$E_thetaleft[sum_k=0^inftyleft(fracn-knright)frac(-1)^kT^kright]=fractheta1+theta$$
Hence by Lehmann-Scheffe theorem, UMVUE of $theta/(1+theta)$ is
beginalign
h_1(T)&=begincasesdisplaystylesum_k=1^inftyleft(fracn+knright)T^k&,text if 0<theta<1\\displaystylesum_k=0^inftyleft(fracn-knright)frac(-1)^kT^k&,text if thetage1 endcases
\\&=begincasesdisplaystylefracT(n+1-nT)n(T-1)^2&,text if 0<theta<1\\displaystylefracT(n+1+nT)n(T+1)^2&,text if thetage1endcases
endalign
However, upon verification of unbiasedness for some values of $n$, it looks like only $$h_1(T)=displaystylefracT(n+1+nT)n(T+1)^2$$ should be the correct answer for all $theta>0$. I am not quite sure why that happens.
For the second problem, we can use the power series expansion of $e^theta$ to obtain
$$E_thetaleft[sum_k=-1^inftyleft(fracn+knright)fracT^k(k+1)!right]=sum_j=0^infty fractheta^j-1j!=frace^thetatheta$$
So the UMVUE of $e^theta/theta$ is
beginalign
h_2(T)&=sum_k=-1^inftyleft(fracn+knright)fracT^k(k+1)!
\\&=frace^T(n-1+T)nT
endalign
1
ah! I understand! But the problem I am getting is somewhere else. Take $k=-n-2$. Then: $$E(T^-n-2)=fracntheta^nint_0^thetat^-n-2+n-1dt=fracntheta^nint_0^thetat^-3dt=fracntheta^nint_0^thetafrac1t^3dt$$ I can not integrate this. That is the problem.
– Stat_prob_001
Aug 23 at 10:11
2
@StubbornAtom, I think the OP meant to say that the integration $int_0^theta frac1t^3dt$ is undefined at $0$, you can not integrate that thing in the first place.
– Shanks
Aug 23 at 16:11
1
@Stat_prob_001 Do have a look at my edit.
– StubbornAtom
Aug 24 at 17:48
1
@StubbornAtom thanks for the update. This is definitely a very useful method. But, just for curiosity, I tried to use the that method to find UMVUE of $theta^-n-2$, and it came out to be $-frac2nT^-n-2$. But $E(-frac2nT^-n-2)$ do not exists. So, I think (maybe), this method can only be used if UMVUE of a function $theta$ exists for all $theta$. So, it is needed to prove that UMVUE of $fractheta1+theta$ actually exists for all $theta$. Is there is any way to establish such think? I mean how to prove UMVUE of $fractheta1+theta$ exists?
– Stat_prob_001
Aug 25 at 8:12
1
@Stat_prob_001 There is a theorem that says a necessary and sufficient condition for an unbiased estimator to be the UMVUE of a given parametric function is that it must be uncorrelated with every unbiased estimator of zero. This result can be used to inspect whether UMVUE for a parametric function exists or not.
– StubbornAtom
Aug 25 at 8:26
 |Â
show 4 more comments
up vote
5
down vote
accepted
You have a $U(-theta,theta)$ population where $thetainmathbb R^+$.
Joint density of the sample $mathbf X=(X_1,X_2,ldots,X_n)$ is
beginalign
f_theta(mathbf x)&=frac1(2theta)^nmathbf1_-theta < x_1, ldots, x_n < theta
\&=frac1(2theta)^nmathbf1_x_1
\&=frac1(2theta)^nmathbf1_max_1le ile n
endalign
It is clear from Factorization theorem that a sufficient statistic for $theta$ is $$T(mathbf X)=max_1le ile n|X_i|$$
One could verify that $|X_i|sim U(0,theta)$, so that the density of $T$ is $$g_theta(t)=fracntheta^nt^n-1mathbf1_0<t<theta$$
That $T$ is a complete statistic for $theta$ is well-known.
We simply have to find unbiased estimators of the parametric functions of $theta$ based on the complete sufficient statistic. This would give us the UMVUE by the Lehmann-Scheffe theorem.
As the support of the complete sufficient statistic here depends on the parameter $theta$, unbiased estimators can be directly obtained through differentiation.
Let $h_1(T)$ and $h_2(T)$ be unbiased estimators of $theta/(1+theta)$ and $e^theta/theta$ respectively, based on the complete sufficient statistic $T$.
That is, for all $theta>0$,
beginalign
qquadquadfracntheta^nint_0^thetah_1(t)t^n-1,dt&=fractheta1+theta
\implies int_0^thetah_1(t)t^n-1,dt &= fractheta^n+1n(1+theta)
endalign
Differentiating both sides wrt $theta$,
beginalign
h_1(theta)theta^n-1&=fractheta^n(ntheta+n+1)n(1+theta)^2
\implies h_1(theta) &=fractheta(ntheta+n+1)n(1+theta)^2
endalign
Hence, $$h_1(T)=fracT(nT+n+1)n(1+T)^2$$
Similarly for the second problem, for all $theta>0$,
beginalign
qquadquadfracntheta^nint_0^thetah_2(t)t^n-1,dt&=frace^thetatheta
\implies int_0^thetah_2(t)t^n-1,dt &= fractheta^n-1 e^thetan
endalign
Differentiating both sides wrt $theta$ yields
beginalign
h_2(theta)theta^n-1&=frace^thetatheta^n-2(theta+n-1)n
\implies h_2(theta) &=frace^theta(theta+n-1)ntheta
endalign
So, $$h_2(T)=frace^T(T+n-1)nT$$
In my initial answer, the following calculation for the UMVUE was rather unnecessary and complicated. Had the support not depended on the parameter, I might have tried this. I am keeping this part in the answer as I might be able to salvage the somewhat faulty argument on some further consideration :
For $k> -n$, we have
beginalign
E_theta(T^k)&=fracntheta^nint_0^theta t^k+n-1,dt\[8pt]
& = fracntheta^kn+k
endalign
This suggests that an unbiased estimator of $theta^k$ based on $T$ is $$left(fracn+knright)T^k$$
For the first problem, one could write
beginalign
fractheta1+theta&=
begincasesleft(1+frac1thetaright)^-1=1-frac1theta+frac1theta^2-frac1theta^3+cdots&,text if theta>1\\theta(1+theta+theta^2+cdots)&,text if 0<theta<1endcases
endalign
For $0<theta<1$, we have
$$E_thetaleft[left(fracn+1nright)T+left(fracn+2nright)T^2+cdotsright]=theta+theta^2+cdots$$
Or, $$E_thetaleft[sum_k=1^inftyleft(fracn+knright)T^kright]=fractheta1+theta$$
For $theta>1$,
$$E_thetaleft[1-left(fracn-1nright)frac1T+left(fracn-2nright)frac1T^2-cdotsright]=1-frac1theta+frac1theta^2-cdots$$
That is, $$E_thetaleft[sum_k=0^inftyleft(fracn-knright)frac(-1)^kT^kright]=fractheta1+theta$$
Hence by Lehmann-Scheffe theorem, UMVUE of $theta/(1+theta)$ is
beginalign
h_1(T)&=begincasesdisplaystylesum_k=1^inftyleft(fracn+knright)T^k&,text if 0<theta<1\\displaystylesum_k=0^inftyleft(fracn-knright)frac(-1)^kT^k&,text if thetage1 endcases
\\&=begincasesdisplaystylefracT(n+1-nT)n(T-1)^2&,text if 0<theta<1\\displaystylefracT(n+1+nT)n(T+1)^2&,text if thetage1endcases
endalign
However, upon verification of unbiasedness for some values of $n$, it looks like only $$h_1(T)=displaystylefracT(n+1+nT)n(T+1)^2$$ should be the correct answer for all $theta>0$. I am not quite sure why that happens.
For the second problem, we can use the power series expansion of $e^theta$ to obtain
$$E_thetaleft[sum_k=-1^inftyleft(fracn+knright)fracT^k(k+1)!right]=sum_j=0^infty fractheta^j-1j!=frace^thetatheta$$
So the UMVUE of $e^theta/theta$ is
beginalign
h_2(T)&=sum_k=-1^inftyleft(fracn+knright)fracT^k(k+1)!
\\&=frace^T(n-1+T)nT
endalign
1
ah! I understand! But the problem I am getting is somewhere else. Take $k=-n-2$. Then: $$E(T^-n-2)=fracntheta^nint_0^thetat^-n-2+n-1dt=fracntheta^nint_0^thetat^-3dt=fracntheta^nint_0^thetafrac1t^3dt$$ I can not integrate this. That is the problem.
– Stat_prob_001
Aug 23 at 10:11
2
@StubbornAtom, I think the OP meant to say that the integration $int_0^theta frac1t^3dt$ is undefined at $0$, you can not integrate that thing in the first place.
– Shanks
Aug 23 at 16:11
1
@Stat_prob_001 Do have a look at my edit.
– StubbornAtom
Aug 24 at 17:48
1
@StubbornAtom thanks for the update. This is definitely a very useful method. But, just for curiosity, I tried to use the that method to find UMVUE of $theta^-n-2$, and it came out to be $-frac2nT^-n-2$. But $E(-frac2nT^-n-2)$ do not exists. So, I think (maybe), this method can only be used if UMVUE of a function $theta$ exists for all $theta$. So, it is needed to prove that UMVUE of $fractheta1+theta$ actually exists for all $theta$. Is there is any way to establish such think? I mean how to prove UMVUE of $fractheta1+theta$ exists?
– Stat_prob_001
Aug 25 at 8:12
1
@Stat_prob_001 There is a theorem that says a necessary and sufficient condition for an unbiased estimator to be the UMVUE of a given parametric function is that it must be uncorrelated with every unbiased estimator of zero. This result can be used to inspect whether UMVUE for a parametric function exists or not.
– StubbornAtom
Aug 25 at 8:26
 |Â
show 4 more comments
up vote
5
down vote
accepted
up vote
5
down vote
accepted
You have a $U(-theta,theta)$ population where $thetainmathbb R^+$.
Joint density of the sample $mathbf X=(X_1,X_2,ldots,X_n)$ is
beginalign
f_theta(mathbf x)&=frac1(2theta)^nmathbf1_-theta < x_1, ldots, x_n < theta
\&=frac1(2theta)^nmathbf1_x_1
\&=frac1(2theta)^nmathbf1_max_1le ile n
endalign
It is clear from Factorization theorem that a sufficient statistic for $theta$ is $$T(mathbf X)=max_1le ile n|X_i|$$
One could verify that $|X_i|sim U(0,theta)$, so that the density of $T$ is $$g_theta(t)=fracntheta^nt^n-1mathbf1_0<t<theta$$
That $T$ is a complete statistic for $theta$ is well-known.
We simply have to find unbiased estimators of the parametric functions of $theta$ based on the complete sufficient statistic. This would give us the UMVUE by the Lehmann-Scheffe theorem.
As the support of the complete sufficient statistic here depends on the parameter $theta$, unbiased estimators can be directly obtained through differentiation.
Let $h_1(T)$ and $h_2(T)$ be unbiased estimators of $theta/(1+theta)$ and $e^theta/theta$ respectively, based on the complete sufficient statistic $T$.
That is, for all $theta>0$,
beginalign
qquadquadfracntheta^nint_0^thetah_1(t)t^n-1,dt&=fractheta1+theta
\implies int_0^thetah_1(t)t^n-1,dt &= fractheta^n+1n(1+theta)
endalign
Differentiating both sides wrt $theta$,
beginalign
h_1(theta)theta^n-1&=fractheta^n(ntheta+n+1)n(1+theta)^2
\implies h_1(theta) &=fractheta(ntheta+n+1)n(1+theta)^2
endalign
Hence, $$h_1(T)=fracT(nT+n+1)n(1+T)^2$$
Similarly for the second problem, for all $theta>0$,
beginalign
qquadquadfracntheta^nint_0^thetah_2(t)t^n-1,dt&=frace^thetatheta
\implies int_0^thetah_2(t)t^n-1,dt &= fractheta^n-1 e^thetan
endalign
Differentiating both sides wrt $theta$ yields
beginalign
h_2(theta)theta^n-1&=frace^thetatheta^n-2(theta+n-1)n
\implies h_2(theta) &=frace^theta(theta+n-1)ntheta
endalign
So, $$h_2(T)=frace^T(T+n-1)nT$$
In my initial answer, the following calculation for the UMVUE was rather unnecessary and complicated. Had the support not depended on the parameter, I might have tried this. I am keeping this part in the answer as I might be able to salvage the somewhat faulty argument on some further consideration :
For $k> -n$, we have
beginalign
E_theta(T^k)&=fracntheta^nint_0^theta t^k+n-1,dt\[8pt]
& = fracntheta^kn+k
endalign
This suggests that an unbiased estimator of $theta^k$ based on $T$ is $$left(fracn+knright)T^k$$
For the first problem, one could write
beginalign
fractheta1+theta&=
begincasesleft(1+frac1thetaright)^-1=1-frac1theta+frac1theta^2-frac1theta^3+cdots&,text if theta>1\\theta(1+theta+theta^2+cdots)&,text if 0<theta<1endcases
endalign
For $0<theta<1$, we have
$$E_thetaleft[left(fracn+1nright)T+left(fracn+2nright)T^2+cdotsright]=theta+theta^2+cdots$$
Or, $$E_thetaleft[sum_k=1^inftyleft(fracn+knright)T^kright]=fractheta1+theta$$
For $theta>1$,
$$E_thetaleft[1-left(fracn-1nright)frac1T+left(fracn-2nright)frac1T^2-cdotsright]=1-frac1theta+frac1theta^2-cdots$$
That is, $$E_thetaleft[sum_k=0^inftyleft(fracn-knright)frac(-1)^kT^kright]=fractheta1+theta$$
Hence by Lehmann-Scheffe theorem, UMVUE of $theta/(1+theta)$ is
beginalign
h_1(T)&=begincasesdisplaystylesum_k=1^inftyleft(fracn+knright)T^k&,text if 0<theta<1\\displaystylesum_k=0^inftyleft(fracn-knright)frac(-1)^kT^k&,text if thetage1 endcases
\\&=begincasesdisplaystylefracT(n+1-nT)n(T-1)^2&,text if 0<theta<1\\displaystylefracT(n+1+nT)n(T+1)^2&,text if thetage1endcases
endalign
However, upon verification of unbiasedness for some values of $n$, it looks like only $$h_1(T)=displaystylefracT(n+1+nT)n(T+1)^2$$ should be the correct answer for all $theta>0$. I am not quite sure why that happens.
For the second problem, we can use the power series expansion of $e^theta$ to obtain
$$E_thetaleft[sum_k=-1^inftyleft(fracn+knright)fracT^k(k+1)!right]=sum_j=0^infty fractheta^j-1j!=frace^thetatheta$$
So the UMVUE of $e^theta/theta$ is
beginalign
h_2(T)&=sum_k=-1^inftyleft(fracn+knright)fracT^k(k+1)!
\\&=frace^T(n-1+T)nT
endalign
You have a $U(-theta,theta)$ population where $thetainmathbb R^+$.
Joint density of the sample $mathbf X=(X_1,X_2,ldots,X_n)$ is
beginalign
f_theta(mathbf x)&=frac1(2theta)^nmathbf1_-theta < x_1, ldots, x_n < theta
\&=frac1(2theta)^nmathbf1_x_1
\&=frac1(2theta)^nmathbf1_max_1le ile n
endalign
It is clear from Factorization theorem that a sufficient statistic for $theta$ is $$T(mathbf X)=max_1le ile n|X_i|$$
One could verify that $|X_i|sim U(0,theta)$, so that the density of $T$ is $$g_theta(t)=fracntheta^nt^n-1mathbf1_0<t<theta$$
That $T$ is a complete statistic for $theta$ is well-known.
We simply have to find unbiased estimators of the parametric functions of $theta$ based on the complete sufficient statistic. This would give us the UMVUE by the Lehmann-Scheffe theorem.
As the support of the complete sufficient statistic here depends on the parameter $theta$, unbiased estimators can be directly obtained through differentiation.
Let $h_1(T)$ and $h_2(T)$ be unbiased estimators of $theta/(1+theta)$ and $e^theta/theta$ respectively, based on the complete sufficient statistic $T$.
That is, for all $theta>0$,
beginalign
qquadquadfracntheta^nint_0^thetah_1(t)t^n-1,dt&=fractheta1+theta
\implies int_0^thetah_1(t)t^n-1,dt &= fractheta^n+1n(1+theta)
endalign
Differentiating both sides wrt $theta$,
beginalign
h_1(theta)theta^n-1&=fractheta^n(ntheta+n+1)n(1+theta)^2
\implies h_1(theta) &=fractheta(ntheta+n+1)n(1+theta)^2
endalign
Hence, $$h_1(T)=fracT(nT+n+1)n(1+T)^2$$
Similarly for the second problem, for all $theta>0$,
beginalign
qquadquadfracntheta^nint_0^thetah_2(t)t^n-1,dt&=frace^thetatheta
\implies int_0^thetah_2(t)t^n-1,dt &= fractheta^n-1 e^thetan
endalign
Differentiating both sides wrt $theta$ yields
beginalign
h_2(theta)theta^n-1&=frace^thetatheta^n-2(theta+n-1)n
\implies h_2(theta) &=frace^theta(theta+n-1)ntheta
endalign
So, $$h_2(T)=frace^T(T+n-1)nT$$
In my initial answer, the following calculation for the UMVUE was rather unnecessary and complicated. Had the support not depended on the parameter, I might have tried this. I am keeping this part in the answer as I might be able to salvage the somewhat faulty argument on some further consideration :
For $k> -n$, we have
beginalign
E_theta(T^k)&=fracntheta^nint_0^theta t^k+n-1,dt\[8pt]
& = fracntheta^kn+k
endalign
This suggests that an unbiased estimator of $theta^k$ based on $T$ is $$left(fracn+knright)T^k$$
For the first problem, one could write
beginalign
fractheta1+theta&=
begincasesleft(1+frac1thetaright)^-1=1-frac1theta+frac1theta^2-frac1theta^3+cdots&,text if theta>1\\theta(1+theta+theta^2+cdots)&,text if 0<theta<1endcases
endalign
For $0<theta<1$, we have
$$E_thetaleft[left(fracn+1nright)T+left(fracn+2nright)T^2+cdotsright]=theta+theta^2+cdots$$
Or, $$E_thetaleft[sum_k=1^inftyleft(fracn+knright)T^kright]=fractheta1+theta$$
For $theta>1$,
$$E_thetaleft[1-left(fracn-1nright)frac1T+left(fracn-2nright)frac1T^2-cdotsright]=1-frac1theta+frac1theta^2-cdots$$
That is, $$E_thetaleft[sum_k=0^inftyleft(fracn-knright)frac(-1)^kT^kright]=fractheta1+theta$$
Hence by Lehmann-Scheffe theorem, UMVUE of $theta/(1+theta)$ is
beginalign
h_1(T)&=begincasesdisplaystylesum_k=1^inftyleft(fracn+knright)T^k&,text if 0<theta<1\\displaystylesum_k=0^inftyleft(fracn-knright)frac(-1)^kT^k&,text if thetage1 endcases
\\&=begincasesdisplaystylefracT(n+1-nT)n(T-1)^2&,text if 0<theta<1\\displaystylefracT(n+1+nT)n(T+1)^2&,text if thetage1endcases
endalign
However, upon verification of unbiasedness for some values of $n$, it looks like only $$h_1(T)=displaystylefracT(n+1+nT)n(T+1)^2$$ should be the correct answer for all $theta>0$. I am not quite sure why that happens.
For the second problem, we can use the power series expansion of $e^theta$ to obtain
$$E_thetaleft[sum_k=-1^inftyleft(fracn+knright)fracT^k(k+1)!right]=sum_j=0^infty fractheta^j-1j!=frace^thetatheta$$
So the UMVUE of $e^theta/theta$ is
beginalign
h_2(T)&=sum_k=-1^inftyleft(fracn+knright)fracT^k(k+1)!
\\&=frace^T(n-1+T)nT
endalign
edited Aug 24 at 17:46
answered Aug 21 at 20:42


StubbornAtom
3,97311135
3,97311135
1
ah! I understand! But the problem I am getting is somewhere else. Take $k=-n-2$. Then: $$E(T^-n-2)=fracntheta^nint_0^thetat^-n-2+n-1dt=fracntheta^nint_0^thetat^-3dt=fracntheta^nint_0^thetafrac1t^3dt$$ I can not integrate this. That is the problem.
– Stat_prob_001
Aug 23 at 10:11
2
@StubbornAtom, I think the OP meant to say that the integration $int_0^theta frac1t^3dt$ is undefined at $0$, you can not integrate that thing in the first place.
– Shanks
Aug 23 at 16:11
1
@Stat_prob_001 Do have a look at my edit.
– StubbornAtom
Aug 24 at 17:48
1
@StubbornAtom thanks for the update. This is definitely a very useful method. But, just for curiosity, I tried to use the that method to find UMVUE of $theta^-n-2$, and it came out to be $-frac2nT^-n-2$. But $E(-frac2nT^-n-2)$ do not exists. So, I think (maybe), this method can only be used if UMVUE of a function $theta$ exists for all $theta$. So, it is needed to prove that UMVUE of $fractheta1+theta$ actually exists for all $theta$. Is there is any way to establish such think? I mean how to prove UMVUE of $fractheta1+theta$ exists?
– Stat_prob_001
Aug 25 at 8:12
1
@Stat_prob_001 There is a theorem that says a necessary and sufficient condition for an unbiased estimator to be the UMVUE of a given parametric function is that it must be uncorrelated with every unbiased estimator of zero. This result can be used to inspect whether UMVUE for a parametric function exists or not.
– StubbornAtom
Aug 25 at 8:26
 |Â
show 4 more comments
1
ah! I understand! But the problem I am getting is somewhere else. Take $k=-n-2$. Then: $$E(T^-n-2)=fracntheta^nint_0^thetat^-n-2+n-1dt=fracntheta^nint_0^thetat^-3dt=fracntheta^nint_0^thetafrac1t^3dt$$ I can not integrate this. That is the problem.
– Stat_prob_001
Aug 23 at 10:11
2
@StubbornAtom, I think the OP meant to say that the integration $int_0^theta frac1t^3dt$ is undefined at $0$, you can not integrate that thing in the first place.
– Shanks
Aug 23 at 16:11
1
@Stat_prob_001 Do have a look at my edit.
– StubbornAtom
Aug 24 at 17:48
1
@StubbornAtom thanks for the update. This is definitely a very useful method. But, just for curiosity, I tried to use the that method to find UMVUE of $theta^-n-2$, and it came out to be $-frac2nT^-n-2$. But $E(-frac2nT^-n-2)$ do not exists. So, I think (maybe), this method can only be used if UMVUE of a function $theta$ exists for all $theta$. So, it is needed to prove that UMVUE of $fractheta1+theta$ actually exists for all $theta$. Is there is any way to establish such think? I mean how to prove UMVUE of $fractheta1+theta$ exists?
– Stat_prob_001
Aug 25 at 8:12
1
@Stat_prob_001 There is a theorem that says a necessary and sufficient condition for an unbiased estimator to be the UMVUE of a given parametric function is that it must be uncorrelated with every unbiased estimator of zero. This result can be used to inspect whether UMVUE for a parametric function exists or not.
– StubbornAtom
Aug 25 at 8:26
1
1
ah! I understand! But the problem I am getting is somewhere else. Take $k=-n-2$. Then: $$E(T^-n-2)=fracntheta^nint_0^thetat^-n-2+n-1dt=fracntheta^nint_0^thetat^-3dt=fracntheta^nint_0^thetafrac1t^3dt$$ I can not integrate this. That is the problem.
– Stat_prob_001
Aug 23 at 10:11
ah! I understand! But the problem I am getting is somewhere else. Take $k=-n-2$. Then: $$E(T^-n-2)=fracntheta^nint_0^thetat^-n-2+n-1dt=fracntheta^nint_0^thetat^-3dt=fracntheta^nint_0^thetafrac1t^3dt$$ I can not integrate this. That is the problem.
– Stat_prob_001
Aug 23 at 10:11
2
2
@StubbornAtom, I think the OP meant to say that the integration $int_0^theta frac1t^3dt$ is undefined at $0$, you can not integrate that thing in the first place.
– Shanks
Aug 23 at 16:11
@StubbornAtom, I think the OP meant to say that the integration $int_0^theta frac1t^3dt$ is undefined at $0$, you can not integrate that thing in the first place.
– Shanks
Aug 23 at 16:11
1
1
@Stat_prob_001 Do have a look at my edit.
– StubbornAtom
Aug 24 at 17:48
@Stat_prob_001 Do have a look at my edit.
– StubbornAtom
Aug 24 at 17:48
1
1
@StubbornAtom thanks for the update. This is definitely a very useful method. But, just for curiosity, I tried to use the that method to find UMVUE of $theta^-n-2$, and it came out to be $-frac2nT^-n-2$. But $E(-frac2nT^-n-2)$ do not exists. So, I think (maybe), this method can only be used if UMVUE of a function $theta$ exists for all $theta$. So, it is needed to prove that UMVUE of $fractheta1+theta$ actually exists for all $theta$. Is there is any way to establish such think? I mean how to prove UMVUE of $fractheta1+theta$ exists?
– Stat_prob_001
Aug 25 at 8:12
@StubbornAtom thanks for the update. This is definitely a very useful method. But, just for curiosity, I tried to use the that method to find UMVUE of $theta^-n-2$, and it came out to be $-frac2nT^-n-2$. But $E(-frac2nT^-n-2)$ do not exists. So, I think (maybe), this method can only be used if UMVUE of a function $theta$ exists for all $theta$. So, it is needed to prove that UMVUE of $fractheta1+theta$ actually exists for all $theta$. Is there is any way to establish such think? I mean how to prove UMVUE of $fractheta1+theta$ exists?
– Stat_prob_001
Aug 25 at 8:12
1
1
@Stat_prob_001 There is a theorem that says a necessary and sufficient condition for an unbiased estimator to be the UMVUE of a given parametric function is that it must be uncorrelated with every unbiased estimator of zero. This result can be used to inspect whether UMVUE for a parametric function exists or not.
– StubbornAtom
Aug 25 at 8:26
@Stat_prob_001 There is a theorem that says a necessary and sufficient condition for an unbiased estimator to be the UMVUE of a given parametric function is that it must be uncorrelated with every unbiased estimator of zero. This result can be used to inspect whether UMVUE for a parametric function exists or not.
– StubbornAtom
Aug 25 at 8:26
 |Â
show 4 more comments
Sign up or log in
StackExchange.ready(function ()
StackExchange.helpers.onClickDraftSave('#login-link');
);
Sign up using Google
Sign up using Facebook
Sign up using Email and Password
Post as a guest
StackExchange.ready(
function ()
StackExchange.openid.initPostLogin('.new-post-login', 'https%3a%2f%2fmath.stackexchange.com%2fquestions%2f2889931%2fumvue-of-frac-theta1-theta-and-frace-theta-theta-from-u-the%23new-answer', 'question_page');
);
Post as a guest
Sign up or log in
StackExchange.ready(function ()
StackExchange.helpers.onClickDraftSave('#login-link');
);
Sign up using Google
Sign up using Facebook
Sign up using Email and Password
Post as a guest
Sign up or log in
StackExchange.ready(function ()
StackExchange.helpers.onClickDraftSave('#login-link');
);
Sign up using Google
Sign up using Facebook
Sign up using Email and Password
Post as a guest
Sign up or log in
StackExchange.ready(function ()
StackExchange.helpers.onClickDraftSave('#login-link');
);
Sign up using Google
Sign up using Facebook
Sign up using Email and Password
Sign up using Google
Sign up using Facebook
Sign up using Email and Password
1
A complete sufficient statistic for $theta$ is simply $max |X_i|$. Did you try finding the unbiased estimators of i) and ii) ?
– StubbornAtom
Aug 21 at 16:04
@StubbornAtom I wrote that I could not find u.e.. I am asking for a way (or a hint) to find u.e.
– Stat_prob_001
Aug 21 at 16:09
Regarding i), let $g(theta)=fractheta1+theta$, where $theta$ is obviously positive. If $theta>1$, then one could write $$g(theta)=left(1+frac1thetaright)^-1=1-frac1theta+frac1theta^2-frac1theta^3+cdots$$ If $0<theta<1$, then $$g(theta)=theta(1+theta+theta^2+cdots)$$...
– StubbornAtom
Aug 21 at 17:11
...So if one could find unbiased estimators of the form $theta^k$ or $1/theta^k$, then combining them he could get an unbiased estimator $T$ (say) of $g(theta)$. By Lehmann-Scheffe theorem, $E(Tmid max|X_i|)$ would be the UMVUE of $g(theta)$. Note that $X_isim U(-theta,theta)implies|X_i|sim U(0,theta)$ and $max |X_i|$ is a complete sufficient statistic for the family. This is just a thought, since ultimately an unbiased estimator of $g(theta)$ based on $max |X_i|$ would be enough for the final answer.
– StubbornAtom
Aug 21 at 17:11
@StubbornAtom very much thank you. Although I could not find any estimator of $1/theta^k$, for $kgeq n$. Is it even possible to find unbiased estimator??
– Stat_prob_001
Aug 21 at 18:53