Convex optimisation with nonconvex constraint function
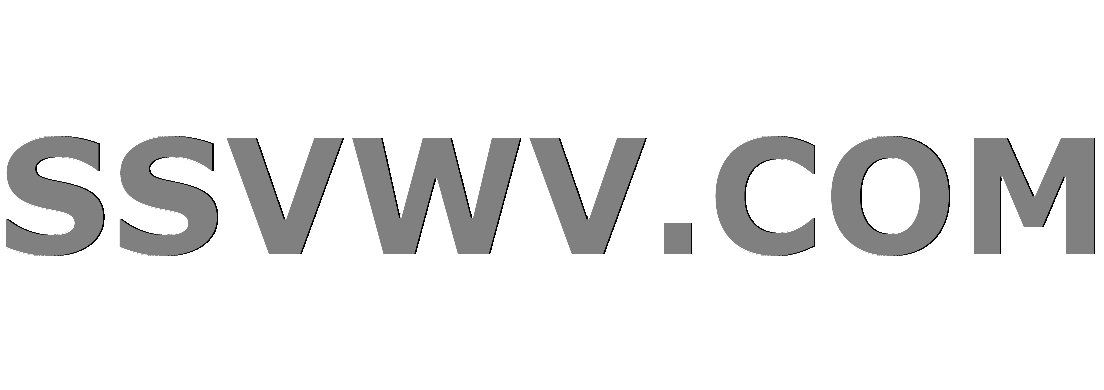
Multi tool use
Clash Royale CLAN TAG#URR8PPP
up vote
0
down vote
favorite
May I ask for a convex optimisation problem (convex/concave objective with convex feasible region), if some of the constraint function is not convex, is kkt still sufficient and necessary for optimality (under slater condition)?
optimization convex-optimization non-convex-optimization
 |Â
show 1 more comment
up vote
0
down vote
favorite
May I ask for a convex optimisation problem (convex/concave objective with convex feasible region), if some of the constraint function is not convex, is kkt still sufficient and necessary for optimality (under slater condition)?
optimization convex-optimization non-convex-optimization
1
With constraint qualification, KKT will be necessary, in general not sufficient.
– user251257
Nov 28 '16 at 20:01
1
The condition "some of the constraint function is not convex" is not sufficient information to rule out a possiblility of rewriting the problem so that it becomes a convex optimization problem. As you seem to point out, the key would be arranging for the feasible reason to be convex (which might or might not be the case when one or more constraint functions are not convex, as stated).
– hardmath
Nov 28 '16 at 20:01
1
If you manage to find a KKT point where no non-convex constraints are active then it is the global minimum.
– A.Γ.
Nov 28 '16 at 20:15
It's not a convex optimization problem if even one of the constraint functions is nonconvex. That is the case even if the feasible region is a convex set.
– Michael Grant
Nov 29 '16 at 3:36
I don't think you can reasonably claim adherence to a Slater condition with a non-convex constraint, at least not in general.
– Michael Grant
Nov 29 '16 at 3:39
 |Â
show 1 more comment
up vote
0
down vote
favorite
up vote
0
down vote
favorite
May I ask for a convex optimisation problem (convex/concave objective with convex feasible region), if some of the constraint function is not convex, is kkt still sufficient and necessary for optimality (under slater condition)?
optimization convex-optimization non-convex-optimization
May I ask for a convex optimisation problem (convex/concave objective with convex feasible region), if some of the constraint function is not convex, is kkt still sufficient and necessary for optimality (under slater condition)?
optimization convex-optimization non-convex-optimization
edited Jul 10 at 6:56
Rodrigo de Azevedo
12.6k41751
12.6k41751
asked Nov 28 '16 at 19:55
user112758
1776
1776
1
With constraint qualification, KKT will be necessary, in general not sufficient.
– user251257
Nov 28 '16 at 20:01
1
The condition "some of the constraint function is not convex" is not sufficient information to rule out a possiblility of rewriting the problem so that it becomes a convex optimization problem. As you seem to point out, the key would be arranging for the feasible reason to be convex (which might or might not be the case when one or more constraint functions are not convex, as stated).
– hardmath
Nov 28 '16 at 20:01
1
If you manage to find a KKT point where no non-convex constraints are active then it is the global minimum.
– A.Γ.
Nov 28 '16 at 20:15
It's not a convex optimization problem if even one of the constraint functions is nonconvex. That is the case even if the feasible region is a convex set.
– Michael Grant
Nov 29 '16 at 3:36
I don't think you can reasonably claim adherence to a Slater condition with a non-convex constraint, at least not in general.
– Michael Grant
Nov 29 '16 at 3:39
 |Â
show 1 more comment
1
With constraint qualification, KKT will be necessary, in general not sufficient.
– user251257
Nov 28 '16 at 20:01
1
The condition "some of the constraint function is not convex" is not sufficient information to rule out a possiblility of rewriting the problem so that it becomes a convex optimization problem. As you seem to point out, the key would be arranging for the feasible reason to be convex (which might or might not be the case when one or more constraint functions are not convex, as stated).
– hardmath
Nov 28 '16 at 20:01
1
If you manage to find a KKT point where no non-convex constraints are active then it is the global minimum.
– A.Γ.
Nov 28 '16 at 20:15
It's not a convex optimization problem if even one of the constraint functions is nonconvex. That is the case even if the feasible region is a convex set.
– Michael Grant
Nov 29 '16 at 3:36
I don't think you can reasonably claim adherence to a Slater condition with a non-convex constraint, at least not in general.
– Michael Grant
Nov 29 '16 at 3:39
1
1
With constraint qualification, KKT will be necessary, in general not sufficient.
– user251257
Nov 28 '16 at 20:01
With constraint qualification, KKT will be necessary, in general not sufficient.
– user251257
Nov 28 '16 at 20:01
1
1
The condition "some of the constraint function is not convex" is not sufficient information to rule out a possiblility of rewriting the problem so that it becomes a convex optimization problem. As you seem to point out, the key would be arranging for the feasible reason to be convex (which might or might not be the case when one or more constraint functions are not convex, as stated).
– hardmath
Nov 28 '16 at 20:01
The condition "some of the constraint function is not convex" is not sufficient information to rule out a possiblility of rewriting the problem so that it becomes a convex optimization problem. As you seem to point out, the key would be arranging for the feasible reason to be convex (which might or might not be the case when one or more constraint functions are not convex, as stated).
– hardmath
Nov 28 '16 at 20:01
1
1
If you manage to find a KKT point where no non-convex constraints are active then it is the global minimum.
– A.Γ.
Nov 28 '16 at 20:15
If you manage to find a KKT point where no non-convex constraints are active then it is the global minimum.
– A.Γ.
Nov 28 '16 at 20:15
It's not a convex optimization problem if even one of the constraint functions is nonconvex. That is the case even if the feasible region is a convex set.
– Michael Grant
Nov 29 '16 at 3:36
It's not a convex optimization problem if even one of the constraint functions is nonconvex. That is the case even if the feasible region is a convex set.
– Michael Grant
Nov 29 '16 at 3:36
I don't think you can reasonably claim adherence to a Slater condition with a non-convex constraint, at least not in general.
– Michael Grant
Nov 29 '16 at 3:39
I don't think you can reasonably claim adherence to a Slater condition with a non-convex constraint, at least not in general.
– Michael Grant
Nov 29 '16 at 3:39
 |Â
show 1 more comment
1 Answer
1
active
oldest
votes
up vote
0
down vote
So long as the (min) objective function is convex and the feasible region is convex, then the solution is not affected by some (or all) constraints being written in a non-convex form.
The constraints affect the solution's validity only by their description of the feasible region. In particular a non-convex constraint can be added to a problem, and if the new constraint happens to be satisfied on the feasible region already determined by earlier constraints, it does not affect the solution at all.
In other cases one might express a convex feasible region using a non-convex function. For example, we might have constraints:
$$ beginalign* x &ge 0 \
sqrtx &le 2 endalign* $$
Because these constraints define the convex region $x in [0,4]$, the choice to present that feasible region using the non-convex square root function does not invalidate the use of criteria suitable for convex optimization problems. One can in principle always rewrite the constraints that determine a convex region using convex functions.
add a comment |Â
1 Answer
1
active
oldest
votes
1 Answer
1
active
oldest
votes
active
oldest
votes
active
oldest
votes
up vote
0
down vote
So long as the (min) objective function is convex and the feasible region is convex, then the solution is not affected by some (or all) constraints being written in a non-convex form.
The constraints affect the solution's validity only by their description of the feasible region. In particular a non-convex constraint can be added to a problem, and if the new constraint happens to be satisfied on the feasible region already determined by earlier constraints, it does not affect the solution at all.
In other cases one might express a convex feasible region using a non-convex function. For example, we might have constraints:
$$ beginalign* x &ge 0 \
sqrtx &le 2 endalign* $$
Because these constraints define the convex region $x in [0,4]$, the choice to present that feasible region using the non-convex square root function does not invalidate the use of criteria suitable for convex optimization problems. One can in principle always rewrite the constraints that determine a convex region using convex functions.
add a comment |Â
up vote
0
down vote
So long as the (min) objective function is convex and the feasible region is convex, then the solution is not affected by some (or all) constraints being written in a non-convex form.
The constraints affect the solution's validity only by their description of the feasible region. In particular a non-convex constraint can be added to a problem, and if the new constraint happens to be satisfied on the feasible region already determined by earlier constraints, it does not affect the solution at all.
In other cases one might express a convex feasible region using a non-convex function. For example, we might have constraints:
$$ beginalign* x &ge 0 \
sqrtx &le 2 endalign* $$
Because these constraints define the convex region $x in [0,4]$, the choice to present that feasible region using the non-convex square root function does not invalidate the use of criteria suitable for convex optimization problems. One can in principle always rewrite the constraints that determine a convex region using convex functions.
add a comment |Â
up vote
0
down vote
up vote
0
down vote
So long as the (min) objective function is convex and the feasible region is convex, then the solution is not affected by some (or all) constraints being written in a non-convex form.
The constraints affect the solution's validity only by their description of the feasible region. In particular a non-convex constraint can be added to a problem, and if the new constraint happens to be satisfied on the feasible region already determined by earlier constraints, it does not affect the solution at all.
In other cases one might express a convex feasible region using a non-convex function. For example, we might have constraints:
$$ beginalign* x &ge 0 \
sqrtx &le 2 endalign* $$
Because these constraints define the convex region $x in [0,4]$, the choice to present that feasible region using the non-convex square root function does not invalidate the use of criteria suitable for convex optimization problems. One can in principle always rewrite the constraints that determine a convex region using convex functions.
So long as the (min) objective function is convex and the feasible region is convex, then the solution is not affected by some (or all) constraints being written in a non-convex form.
The constraints affect the solution's validity only by their description of the feasible region. In particular a non-convex constraint can be added to a problem, and if the new constraint happens to be satisfied on the feasible region already determined by earlier constraints, it does not affect the solution at all.
In other cases one might express a convex feasible region using a non-convex function. For example, we might have constraints:
$$ beginalign* x &ge 0 \
sqrtx &le 2 endalign* $$
Because these constraints define the convex region $x in [0,4]$, the choice to present that feasible region using the non-convex square root function does not invalidate the use of criteria suitable for convex optimization problems. One can in principle always rewrite the constraints that determine a convex region using convex functions.
answered Nov 30 '16 at 23:04
hardmath
28.2k94693
28.2k94693
add a comment |Â
add a comment |Â
Sign up or log in
StackExchange.ready(function ()
StackExchange.helpers.onClickDraftSave('#login-link');
);
Sign up using Google
Sign up using Facebook
Sign up using Email and Password
Post as a guest
StackExchange.ready(
function ()
StackExchange.openid.initPostLogin('.new-post-login', 'https%3a%2f%2fmath.stackexchange.com%2fquestions%2f2034786%2fconvex-optimisation-with-nonconvex-constraint-function%23new-answer', 'question_page');
);
Post as a guest
Sign up or log in
StackExchange.ready(function ()
StackExchange.helpers.onClickDraftSave('#login-link');
);
Sign up using Google
Sign up using Facebook
Sign up using Email and Password
Post as a guest
Sign up or log in
StackExchange.ready(function ()
StackExchange.helpers.onClickDraftSave('#login-link');
);
Sign up using Google
Sign up using Facebook
Sign up using Email and Password
Post as a guest
Sign up or log in
StackExchange.ready(function ()
StackExchange.helpers.onClickDraftSave('#login-link');
);
Sign up using Google
Sign up using Facebook
Sign up using Email and Password
Sign up using Google
Sign up using Facebook
Sign up using Email and Password
1
With constraint qualification, KKT will be necessary, in general not sufficient.
– user251257
Nov 28 '16 at 20:01
1
The condition "some of the constraint function is not convex" is not sufficient information to rule out a possiblility of rewriting the problem so that it becomes a convex optimization problem. As you seem to point out, the key would be arranging for the feasible reason to be convex (which might or might not be the case when one or more constraint functions are not convex, as stated).
– hardmath
Nov 28 '16 at 20:01
1
If you manage to find a KKT point where no non-convex constraints are active then it is the global minimum.
– A.Γ.
Nov 28 '16 at 20:15
It's not a convex optimization problem if even one of the constraint functions is nonconvex. That is the case even if the feasible region is a convex set.
– Michael Grant
Nov 29 '16 at 3:36
I don't think you can reasonably claim adherence to a Slater condition with a non-convex constraint, at least not in general.
– Michael Grant
Nov 29 '16 at 3:39